Turkish Folk Music Modes are Automatically Identified with Artificial Learning Technologies
Yayın Tarihi | 02 March 2024, Saturday
The aim is to explain the concept of maqam, which is one of the fundamental components of Turkish Folk Music, and to investigate how it can be used to automatically identify different maqams. This research aims to create an original compilation in this field, focusing particularly on Turkish folk melodies.
The main aim of this study is to investigate how artificial learning technologies, which have made a significant impact in the world of technology in recent years, can be used to explain the concept of makam, a fundamental component of Turkish Folk Music, and to automatically identify different makams. This research aims to create an original compilation in this field, focusing particularly on Turkish folk tunes. Additionally, the development of technology-based decision support systems and their foundation as educational materials also constitute an important aspect of this study. This study also stands out as one of the few studies where Turkish Folk Music and artificial intelligence techniques come together.
Within the scope of the research, makam classifications were performed on a dataset using different machine learning models. Also, the Turkish Folk Music compilation created for this study and to be shared publicly was tested in this study with the aim of shedding light on other scientific research. In this way, the effect of the relevant models on makam recognition was evaluated. It was noted that the prepared compilation also constitutes an important resource for future research.
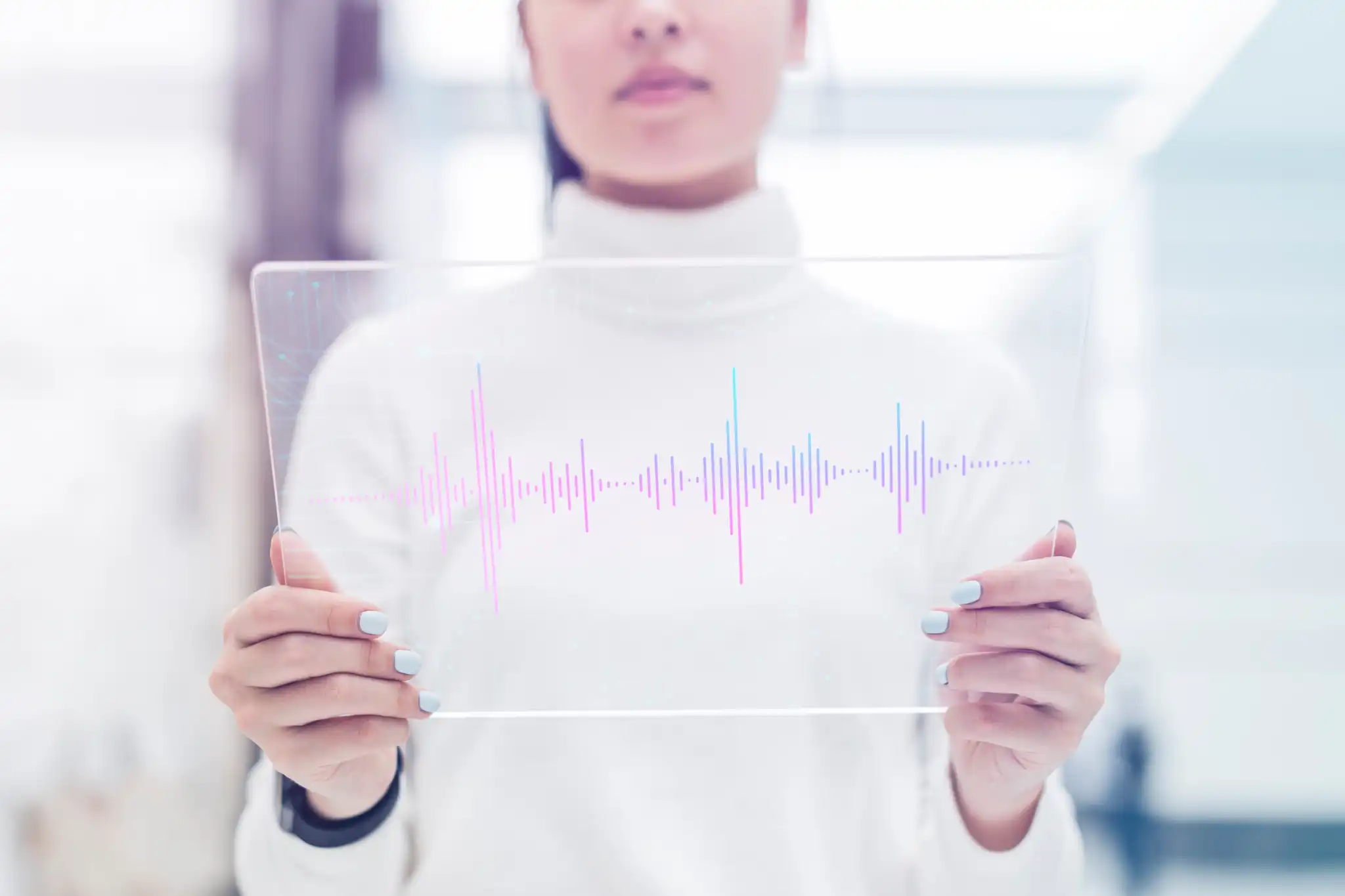
Diğer Haberler
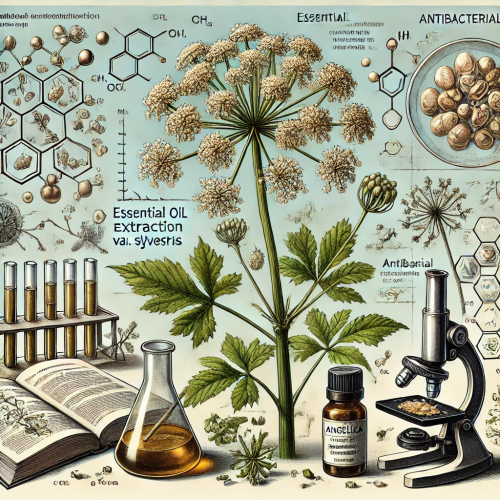
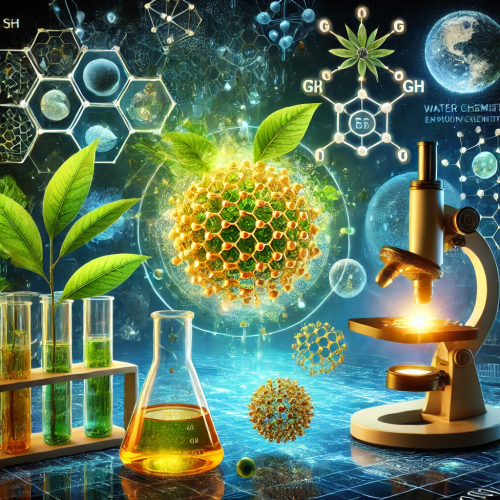
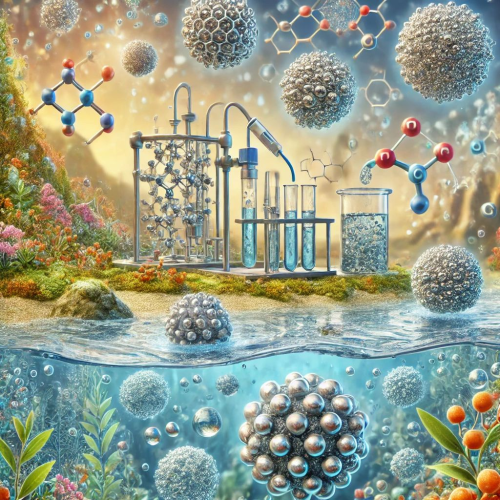
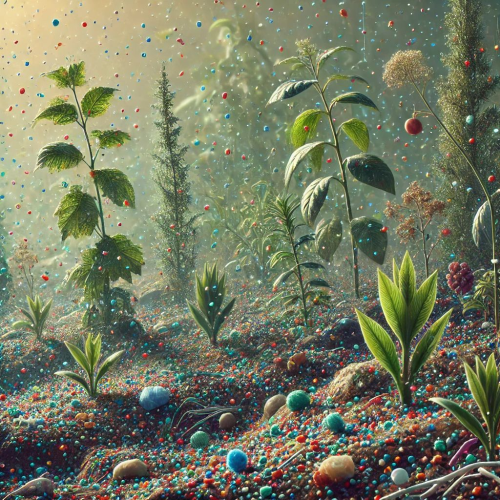
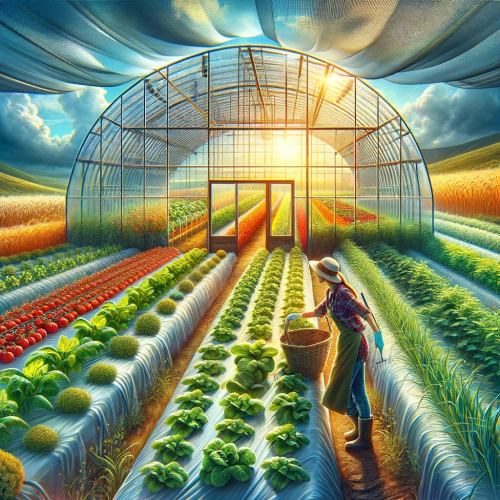
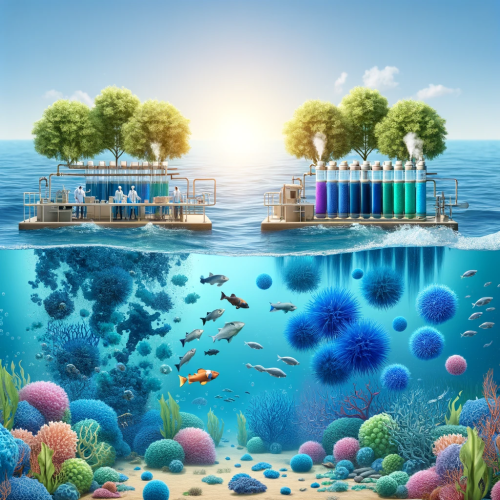
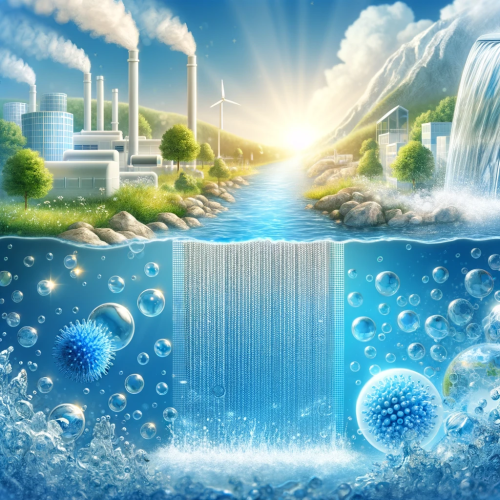
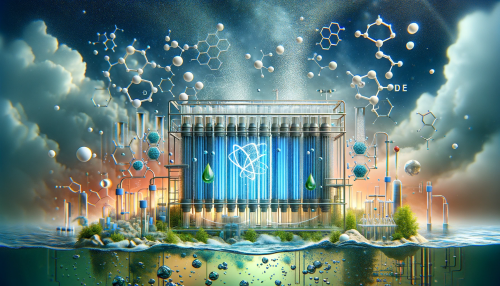
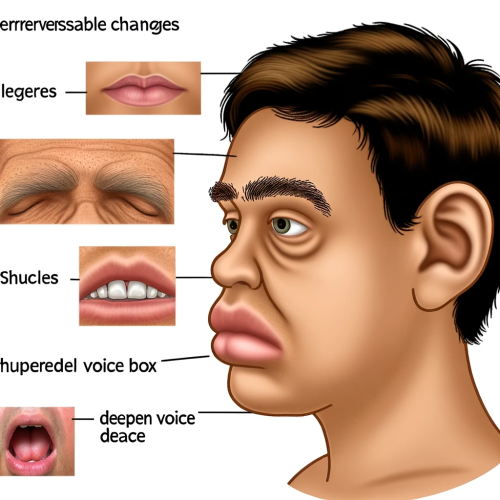
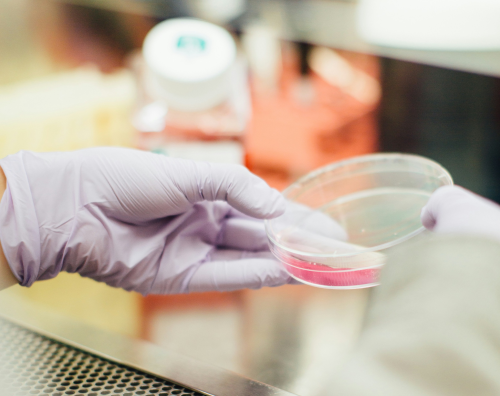